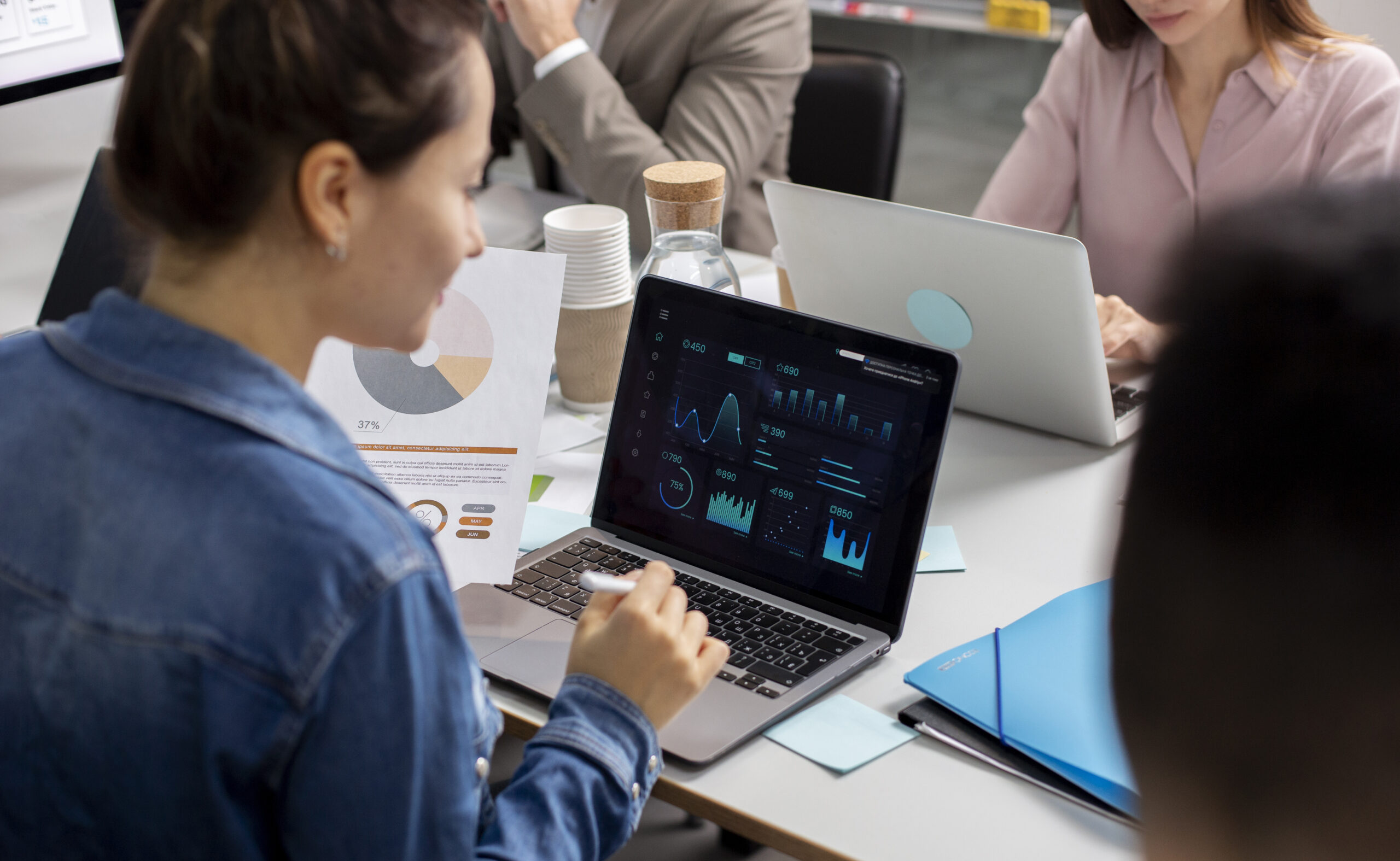
Vector Embeddings: The Secret to Better AI
Efficiently retrieving relevant information from vast datasets is crucial and has multiple industry use cases. Vector embeddings are revolutionizing how we search and interact with data.
What Are Vector Embeddings?
Think of vector embeddings as AI’s way of understanding our language. It’s just like when your partner knows you’re craving an iced latte when you say you’re craving coffee. Vector embeddings are numerical representations of data that capture semantic and contextual meanings. By converting words, phrases, or entire documents into vectors, these embeddings enable machines to understand and process information more naturally and accurately.
Impact of Vector Embeddings
Vector embeddings enhance search systems by enabling semantic understanding beyond traditional keyword matching. Whether you’re managing a knowledge base or trying to improve customer interactions, vector embeddings take the guesswork out of search, making the experience smoother, more intuitive, and a whole lot more satisfying.
Types of Vector Embeddings
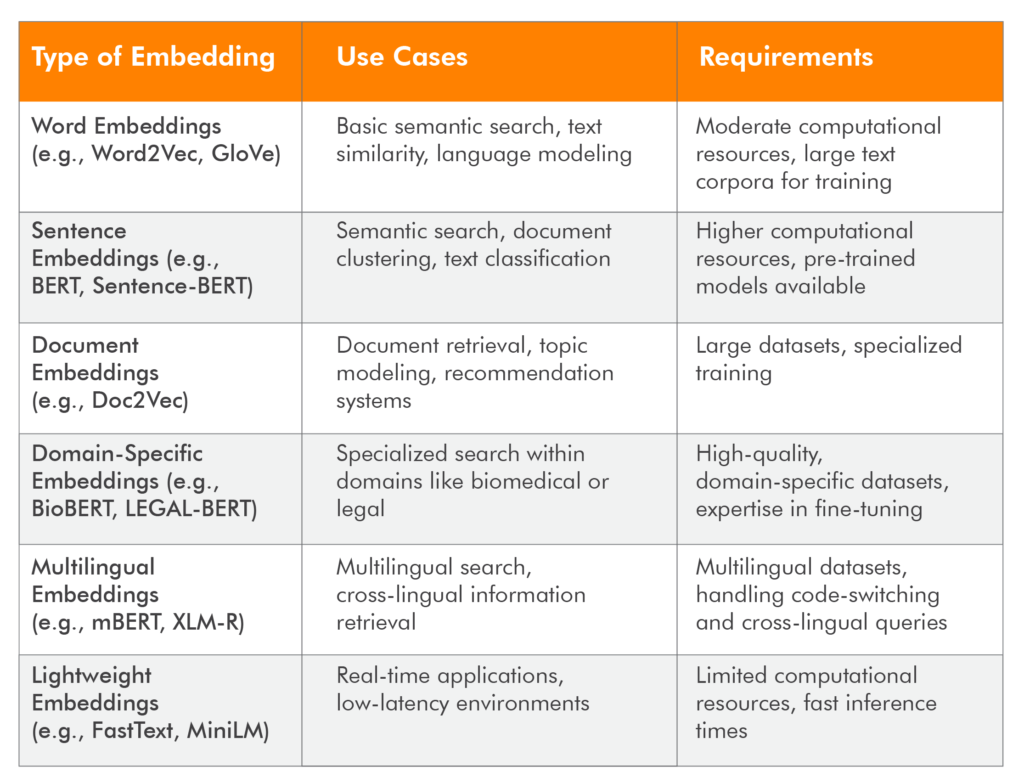
How the Right Vector Embeddings Boosted a Retailer’s Customer Experience
Our client, a Fortune 500 retailer, faced painfully slow data retrieval, causing them to miss out on key customer insights and, ultimately, CX & revenue. It was like trying to find a needle in a haystack, and it was costing them a lot.
After partnering with us, the project team along with the client evaluated the data of client interactions, andselected multilingual embeddings that best suited their specific model & business use case needs.
We helped them deploy optimized vector embeddings, database and retrieval methods transforming their data operations, leading to a 15% increase in annual revenue, a 50% reduction in data processing time, and significantly improved customer interactions. This case highlights how the right data solutions and strategic decision-making can drive substantial business growth and efficiency.
Our Recommendations
Based on our expertise, we recommend the following embedding models:
- For General Use: BERT or Sentence-BERT for balanced accuracy and performance.
- For Specialized Domains: Fine-tuned models like BioBERT or LEGAL-BERT tailored to specific industry needs.
- For Multilingual Applications: mBERT or XLM-R to support diverse linguistic requirements.
- For Limited Resources: FastText or MiniLM for efficient processing and lower computational demands.
Conclusion
We have seen firsthand the transformative power of vector embeddings. From enhancing enterprise search capabilities to revolutionizing e-commerce recommendations, these models enable organizations to unlock new insights and optimize operations.
Vector embeddings are reshaping the way organizations search, access, and leverage information. By representing data as vectors, these models understand the underlying context and semantics, delivering more relevant and useful results than traditional methods.
For more insights on vector embeddings and their application in your industry, you know whom to contact. Together, let’s turn your data into actionable intelligence.