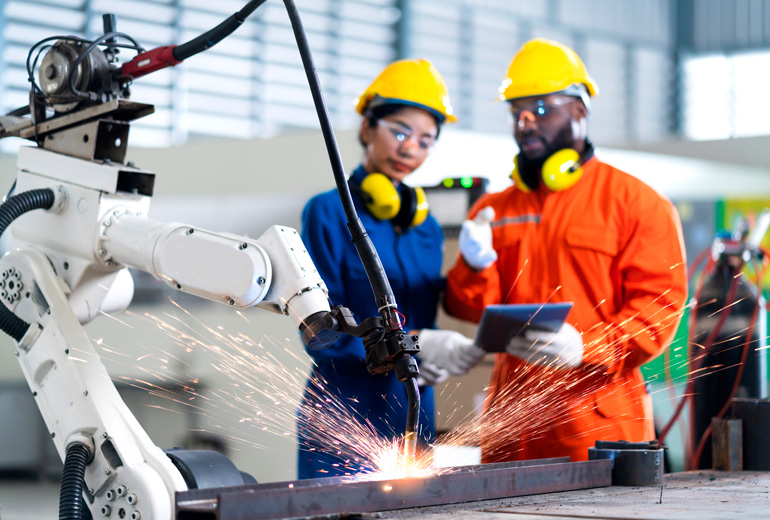
The Key Ingredient of AI Success in Manufacturing – Data Quality!
Manufacturing leaders realize that the potential of AI in their industry is significant for making production and supply chain smoother, faster, and far more efficient. However, the success of AI in manufacturing relies on one crucial factor: data quality. It is projected that by 2025 at least 40%* of manufacturing companies will adopt modern data quality solutions. Read on to understand why Data Quality matters so much.
The Data Spectrum in Manufacturing
In manufacturing, most businesses want to embrace AI for decision-making, but before that understanding how data impacts AI models is paramount.
At the foundational level, data can be fact-based (structured) or opinion-based (semi structured or unstructured). Fact-based data includes quantifiable numbers and specific metrics like production output, material costs, data from sensors, defect rates, or equipment downtime. Opinion-based data includes descriptive elements where interpretive quality is key. These can be feedback from teams on product uality, reasons for unscheduled maintenance and downtime, and usability of new equipment. All this data is unstructured.
As a manufacturing leader, it’s important to know this difference and assess the quality of both kinds of data for planning any AI implementation in the organization. AI can be built on both types of data epending on the business case. Most manufacturing AI use cases rely on structured data, while the quality of unstructured data contributes to improving model efficiency and accuracy.
Data Quality in Manufacturing: Vital for AI Success
Below are the common data quality issues we see in the industry and our recommendations to address them:
- Ensuring reliable inputs for AI systems – Faulty data, be it from human error or system glitches, can result in wrong AI model outputs. In production, if AI is detecting anomalies in production line based on faulty data, it could suggest incorrect actions like ordering unnecessary maintenance or slowing production. Regular data health checks and updates are crucial to ensuring AI models remain reliable and relevant. One of our clients in the pharmaceutical industry used synthetic data for developing an inventory model. The model performed well during the POC phase, but when deployed to production, it met unforeseen issues. We were then brought in to address these challenges. Our data science team analyzed the discrepancy and successfully resolved it by retraining the model with real-world data.
- Addressing data gaps and missing information – Imagine working on an AI model designed to analyze product defects, but the data is missing for 2 major defect types commonly seen in the product. Similarly, in some systems, if orders don’t have an associated customer IDs, AI models might give irrelevant results in such cases. You should consider estimation techniques and algorithms to handle missing data points.
We used this approach in developing a predictive model for a computer vision-based damage estimation solution for an insurance company. We incorporated specific scenarios into the model for which we did not possess real data.
- Choosing impactful data for effective AI outcomes – AI works differently for different types of data as covered earlier. Depending on the business case, make sure to choose the most impactful data. For example, AI can work on production speed data or machine downtime data to improve efficiency. But depending on your purpose (predictive maintenance vs. production optimization), the choice of data will impact the AI model’s success.
- Data standardization across manufacturing systems: Data standardization across systems is key. A factory with multiple production lines might record production output differently across systems. This inconsistency can lead to confusion and errors when analyzing data for AI-based insights. Standardizing data collection processes helps avoid this issue.
While implementing a predictive maintenance solution for a manufacturing company, we met an interesting challenge: a few PLC machines were not compatible for sharing machine data. Our team programmed the PLC using semiconductor logic to make sure that the data from those PLCs was available for analysis. - Real-time data for keeping AI models current: Data can become outdated quickly, especially in fast-moving industries. AI used for supply chain management needs real-time data for demand forecasting and managing inventory. Relying on old inventory data can lead to overstocking or understocking. You should implement real-time data updates and integrations with other systems to ensure your AI has access to the latest information.
One of our clients specifically implemented a Databricks based system to ensure that real-time data was available. This helped us build a real-time alerting system for them. This allowed them to make insightful decisions on the fly, significantly reducing response times.
At Konverge.AI, we recommend all our clients to develop a data-centric AI strategy. Identify operational and process issues caused by poor data quality and address these based on priority. It’s best to have a dedicated data quality team to resolve such problems. This team should have access to the latest tools to help them address data issues. The team should also implement robust data governance best practices to maintain data integrity ensuring long-term success. When in doubt, it’s best to involve subject matter experts to ensure your AI systems perform effectively to generate business value
References:
https://www.gartner.com/en/doc/789057-3-levers-that-manufacturing-cios-must-pull-to-
improve-and-sustain-data-quality